AI apocalypse avoided? Neural networks now smart enough to know when they shouldn’t be trusted
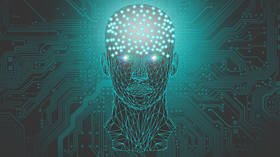
In a development that could scupper the plot of numerous sci-fi movies about an artificial intelligence apocalypse, scientists have created a neural network that is smart enough to know when it shouldn’t be trusted.
With each passing year, artificial intelligence systems known as deep learning neural networks are increasingly being used in areas that could have a massive impact on health and safety, such as transportation and medicine.
The systems are built to aid decision making, and they specialize in weighing up complex datasets that humans simply don’t have the capacity to analyze.
But how do we know their judgement is correct? To get a handle on this problem, the new network can give readings of its confidence level along with its predictions.
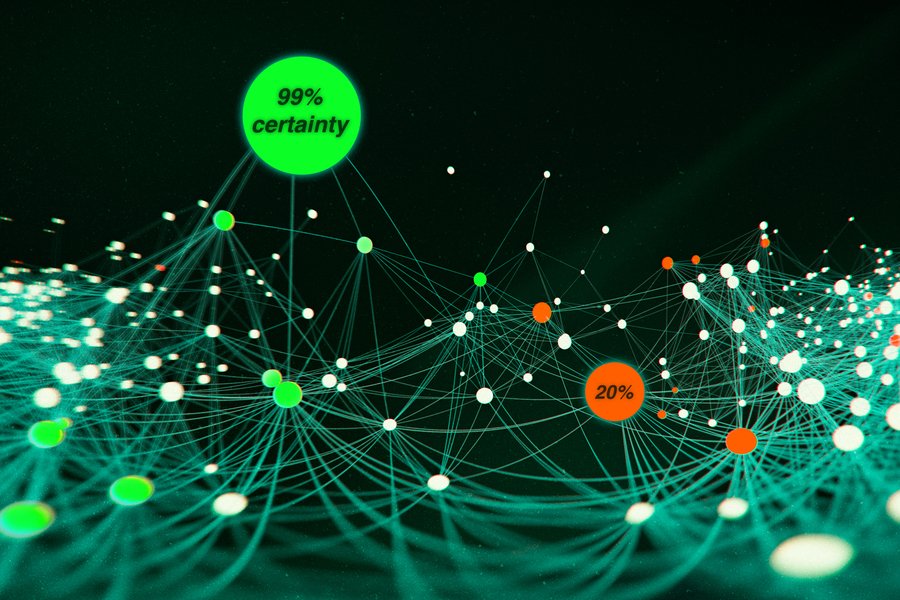
The scientists behind the development say it could save lives, as a system’s level of confidence can be the difference between an autonomous vehicle deciding “it’s all clear to proceed through the intersection” and concluding “it’s probably clear, so stop just in case.”
This self-awareness of trustworthiness feature has been dubbed “deep evidential regression,” and it bases its confidence level on the quality of the available data it has to work with.
The feature improves on previous safeguards by carrying out its analysis without excessive computing demands.
The scientists tested their network by training it to judge depths in different parts of an image, similar to how a self-driving car might calculate proximity to a pedestrian or another vehicle.
Also on rt.com Guardian touts op-ed on why AI takeover won’t happen as ‘written by robot,’ but tech-heads smell a human behind the trickThe system compared well to existing setups, while also having the ability to estimate its own uncertainty. The times the network was least certain were indeed the times it got the depths wrong.
“This idea is important and applicable broadly,” explained one of the researchers, Professor Daniela Rus from the Massachusetts Institute of Technology (MIT).
“It can be used to assess products that rely on learned models. By estimating the uncertainty of a learned model, we also learn how much error to expect from the model, and what missing data could improve the model.”
Like this story? Share it with a friend!